Janelle Shane – You Look Like A Thing And I Love You (2019)
Een boek dat de (on)mogelijkheden van artificial intelligence illustreert aan de hand van grappige voorbeelden. De auteur heeft inmiddels een reputatie opgebouwd op het gebied van ‘AI weirdness’.
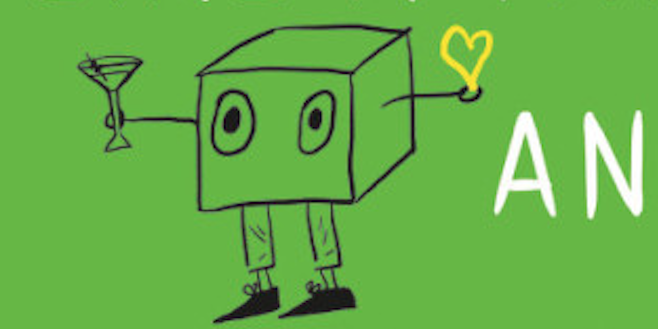
The question many AI’s answer is not ‘What is the best solution?’ but ‘What would the humans have done?’
The key thing about GANs is they’re two algorithms in one, testing each other: the generator tries to imitate the input dataset, the discriminator tries to tell the difference between the generator’s imitation and the real thing.
The narrower the task, the smarter the AI it seems.
If there are problems with the dataset, the algorithm will at best waste time and at worst learn the wrong thing.
Machine learning experts live for moments like this – when the algorithm comes up with a solution that’s both unusual and effective.
Treating a decision as impartial just because it came from an AI is known as math-washing or bias laundering.
"One HR employee for a major technology company recommends slipping the words 'Oxford' or 'Cambridge' into a CV in invisible white text, to pass the automated screening"
– The Guardian
An AI that’s making callbacks to earlier jokes, that sticks with a consistent cast of characters, and that keeps track of the objects in a room probably had a lot of human editing help, at least.
Practical machine learning ends up being a bit of a hybrid between rules-based programming, in which a human tells a computer step-by-step how to solve a problem, and open-ended machine learning, in which an algorithm has to figure everything out.